Stable Diffusion
I took a long time to publish this blog entry. I was sitting with this post half written for weeks now, Most stuff I want to write is out there already, even expressed better than what you will read further but I will publish this nonetheless.
SO what is this new(at the time of writing it was) thing in the town?
Stable Diffusion #
Stable diffusion is a new model from OpenAI that is a successor to Dall-E. The company that trained this model is stability.ai, spent a lot of time and resources to train this model. And more importantly, they released the code and model weights for everyone to use.
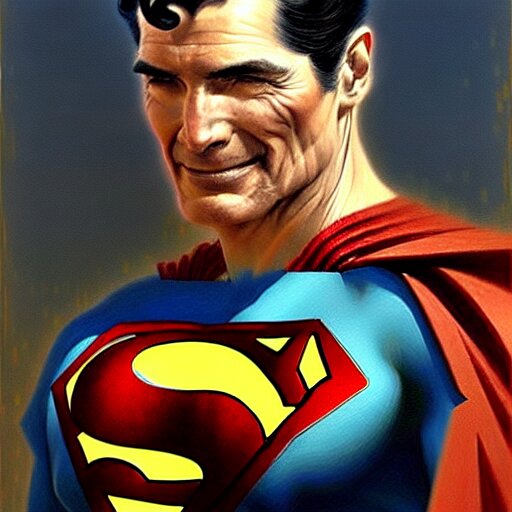
Images posted here are created by Stable Diffusion, on my laptop. Each image is 512x512 pixels. I am using stable-diffusion-ui by cmdr2 to generate these images. Updates were frequent and features are added regularly. It is great and supports a lot of features and my hardware. Took me about 10 to 30 minutes to generate one depending on the number of inference steps.
Because of the log wait times I had my laptop running, generating images with input given from the webUI from my phone all thanks to the tailscale VPN. I was able to generate some really great images. For example, this one.
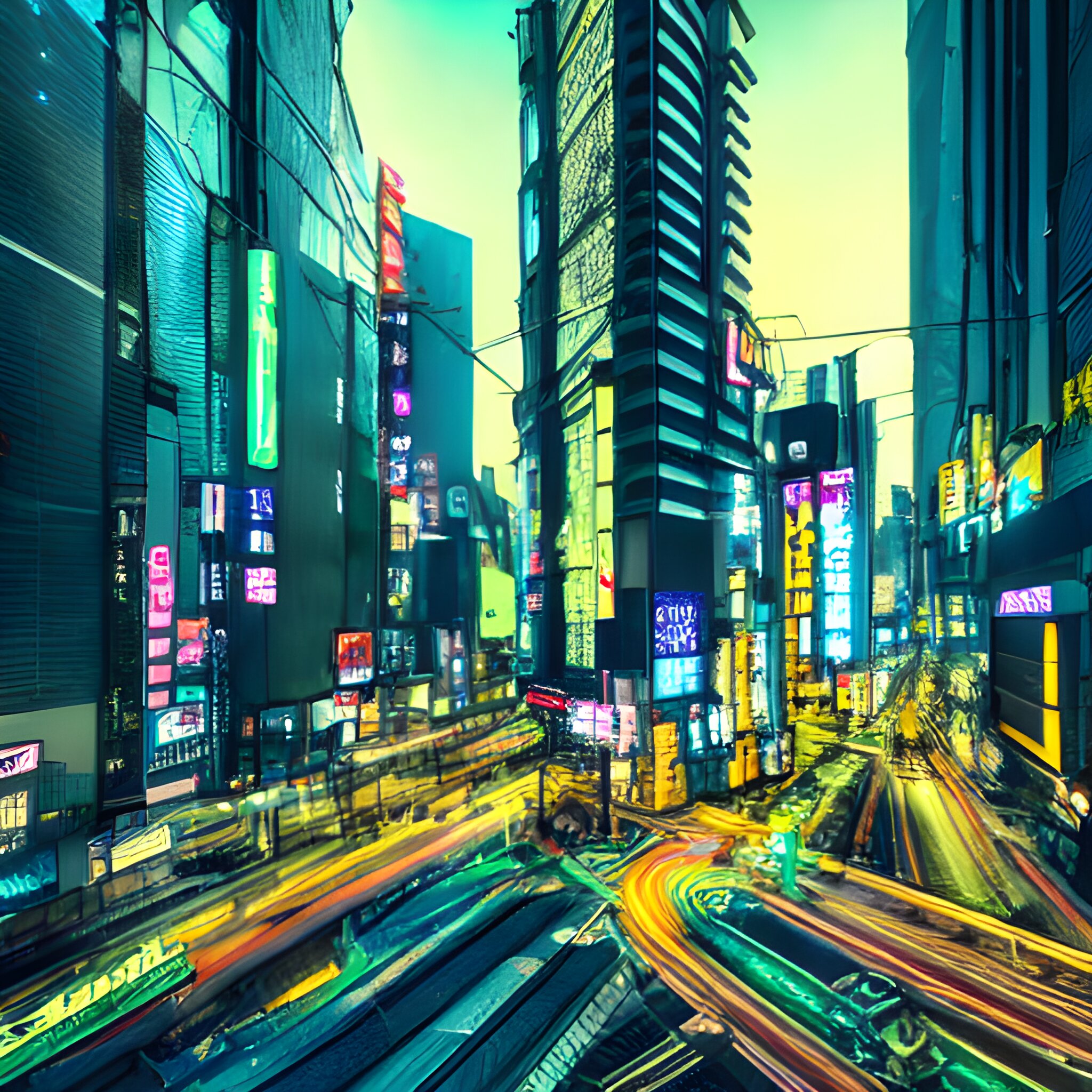
This picture is first generated by stable diffusion and then scaled up to 4k using extended options of stable-diffusion-ui. This just shows how great and extensible open source A.I. related projects can be. It is capable at making great outputs but it is not perfect. Getting desired output is a rare occurrence.
Getting the prompt right is essential most of the time and can be a pain. Even with the right prompt, the output is not always what you want. But with a beefy machine and a lot of time, you can get some really great outputs with iteration.
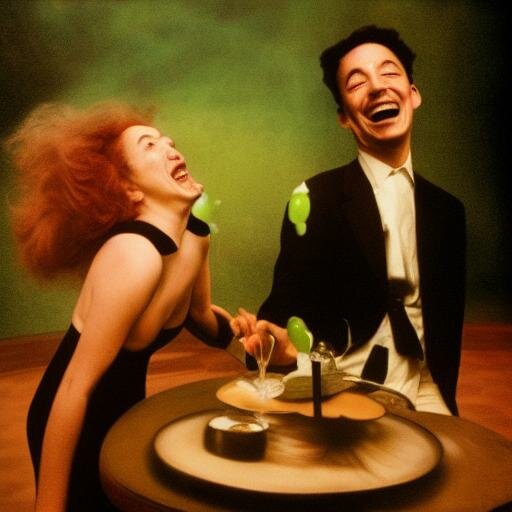
You can get involved with online communities related to generative art and to know how to better frame the prompt. As a developer, you can also contribute to the project and make it better.
But how is it compared to Dall-E?
Comparison with Dall-E #
It is really hard to compare these two models with context. One is built with multi-million dollar budget and the other is built with a fraction of that. But I will try to compare them as much as I can. To be honest difference is not as staggering as I thought it would be.
Prompt #
These both generate images from text and images. Stable diffusion provides much more fine grained control over the process of generating images.
Dall-E is more of a black box. You give it a prompt and it generates an image. And that is it. No way to control or reproduce the output. But what it does for you is pretty amazing. It imagines what you want to see and generates it for you. Unlike stable diffusion, it does not require you to know how to frame the prompt(not to the same extent at least).
In my tests with stable diffusion, image to image was really bad, dealing with abstract(for lack of a better word) idea in image was really bad.
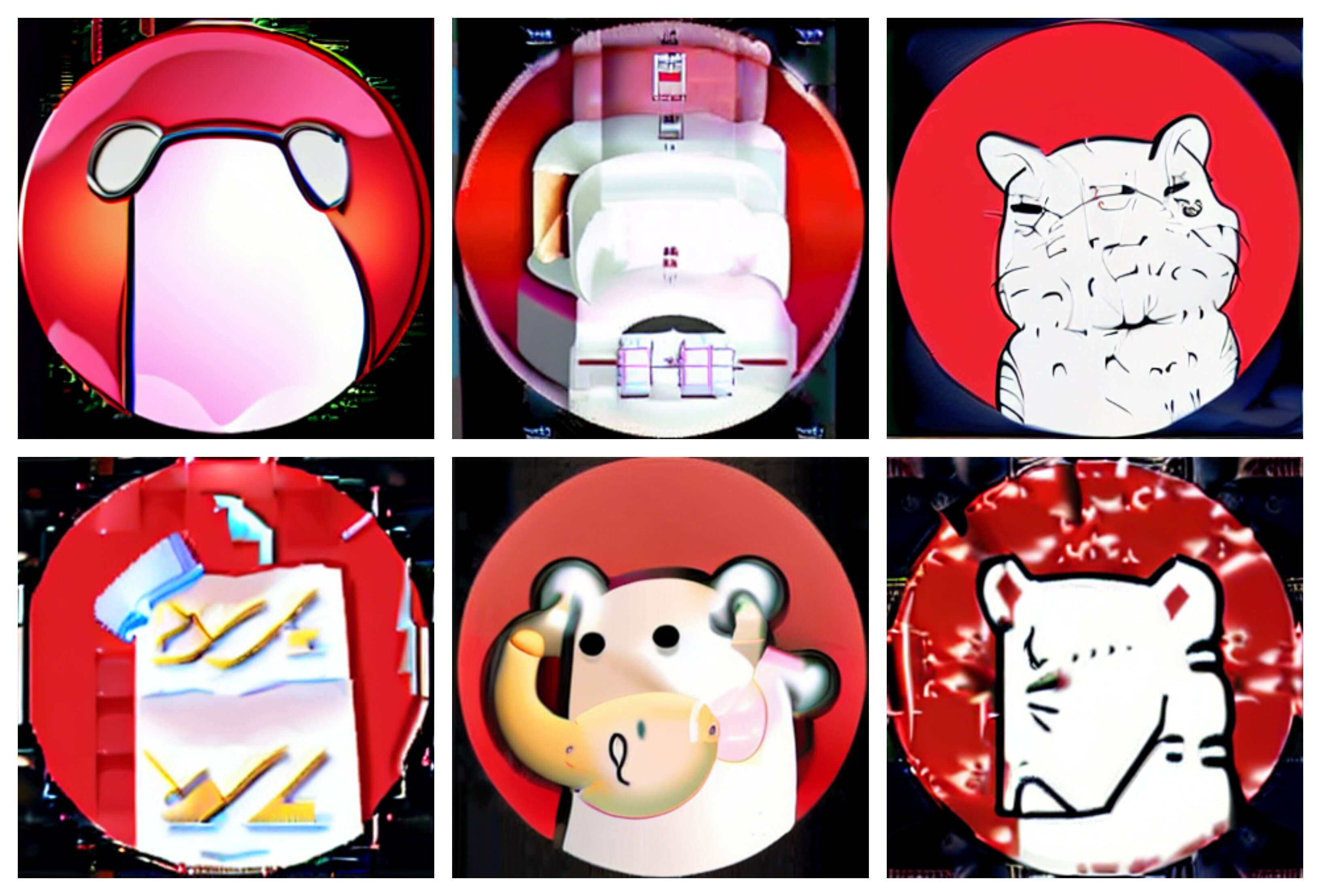
compared to Dall-E
As you can see, stable diffusion is not able to generate a dog from a minimal dog image. This is one of it’s biggest shortcomings.
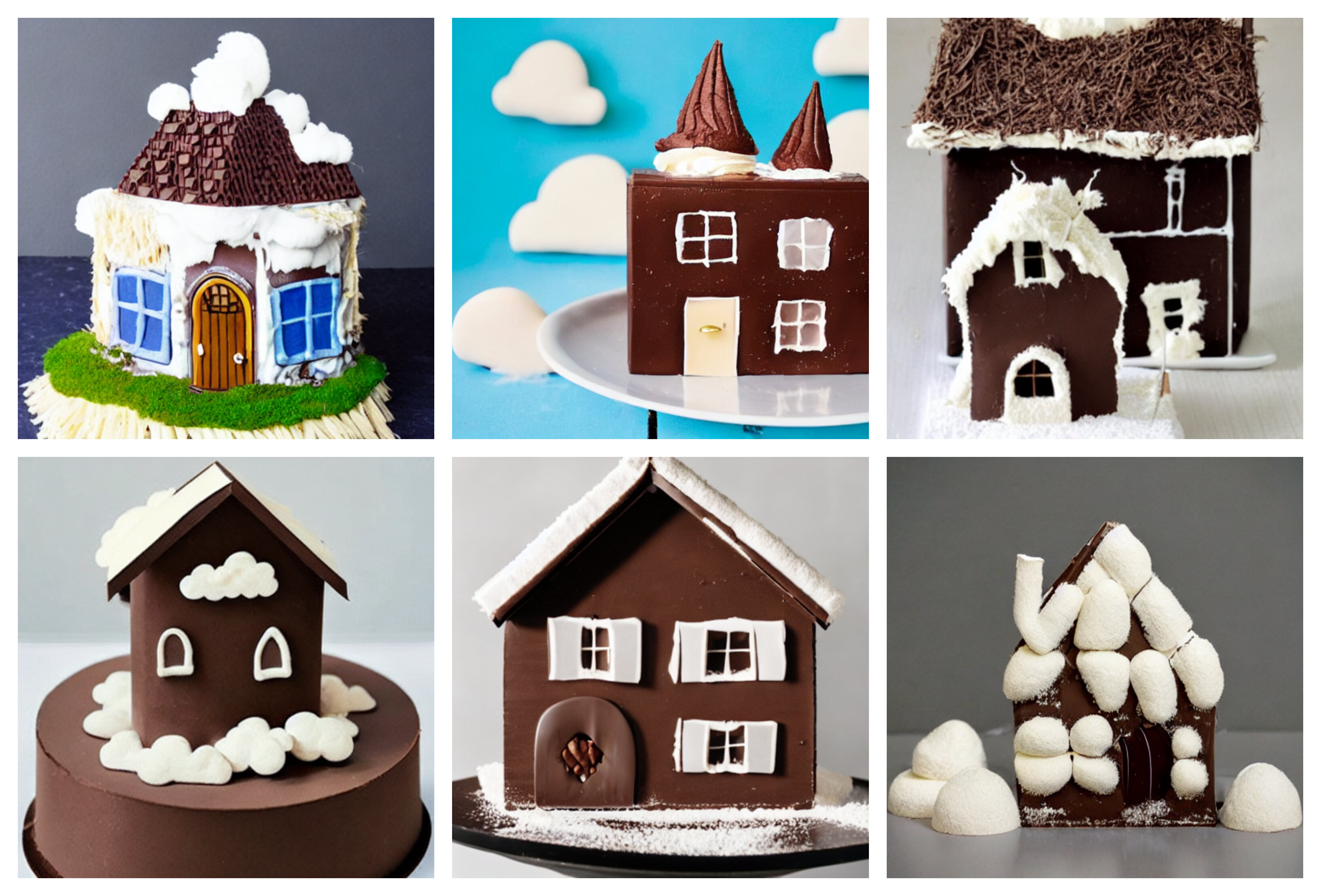
compared to Dall-E
We can see that the stable diffusion produces comparable if not better results than Dall-E here. Dall-E feels much more “creative” than stable diffusion but stable diffusion feels much closer to literal prompt.
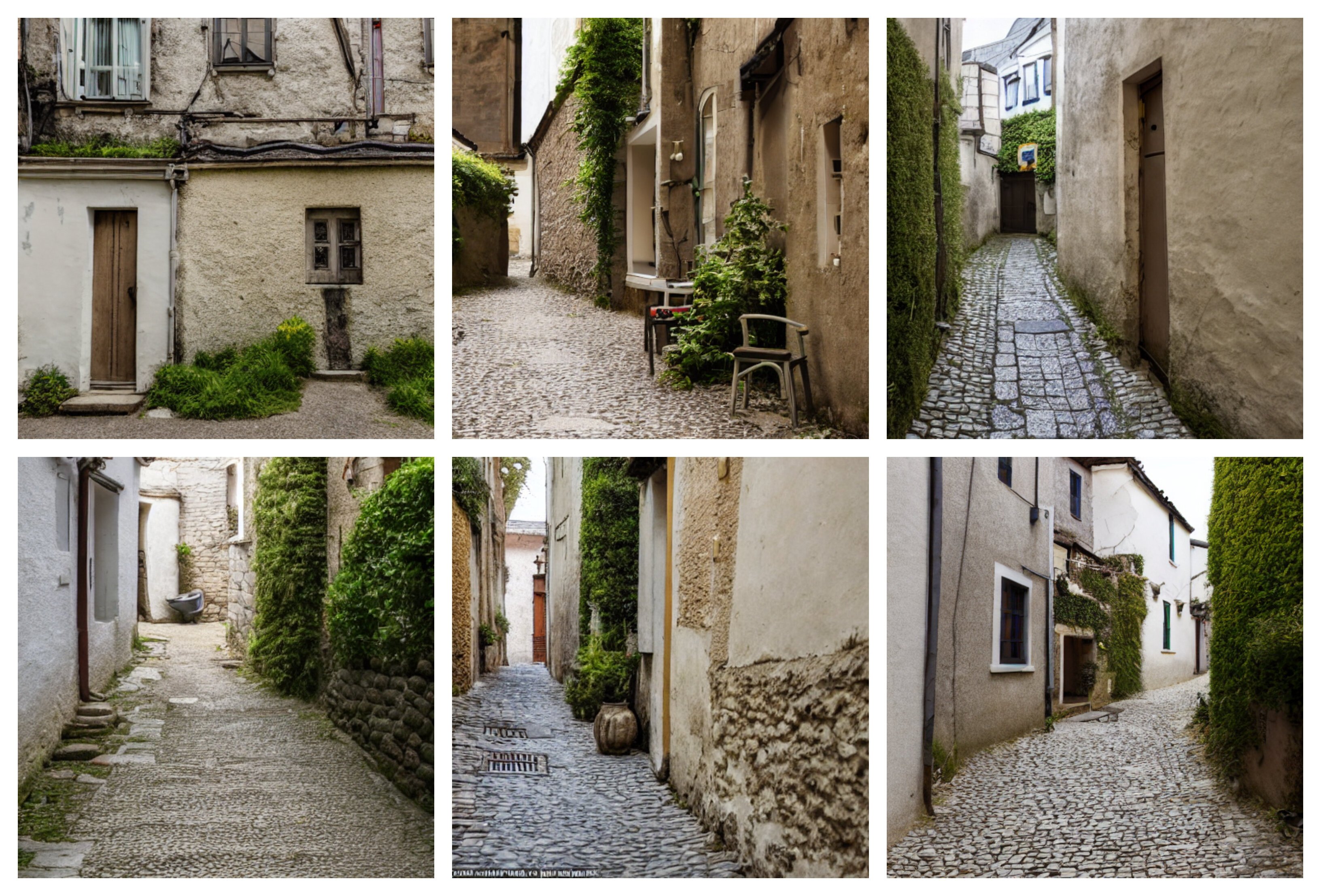
compared to Dall-E
we can see a clear lack in relation between the prompt and the output in stable diffusion. It is not able to generate chairs. Maybe this can be improved with more training or better prompt framing.
With this I’d like to conclude this article. Stable diffusion is a great model and I am really excited to see what the future holds for it. Especially with community involvement and contributions.